Don’t Rush AI Integration in Your SaaS Without Knowing This
- SoftudeApril 29, 2025
- Last Modified onApril 29, 2025
Over the past decade, SaaS companies have relied on incremental feature updates to retain customers and drive growth. Now, AI agent integration represents the next major leap, one that can transform routine tasks into personalized experiences, accelerate decision-making, and unlock efficiencies across your organization. But embedding an AI agent isn’t as simple as wiring up a new API or checking a box on your roadmap. We tell you what every decision-maker must address before integrating an AI agent into a SaaS product.
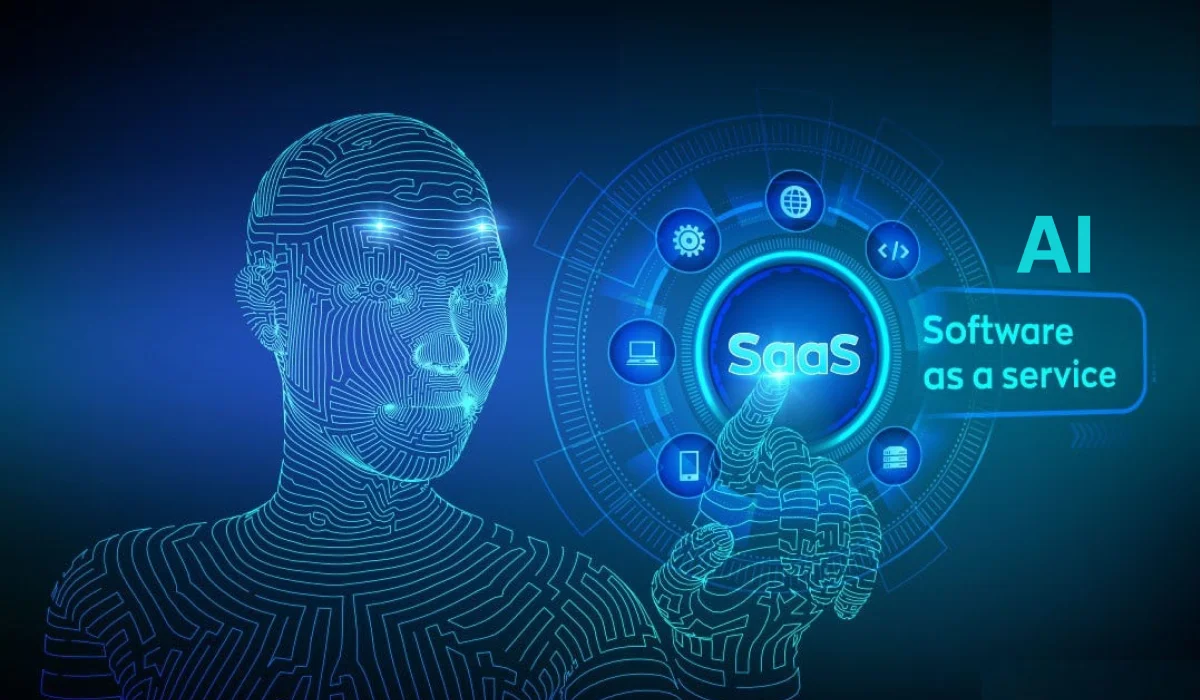
3 Key Areas Where You Must Focus for Successful AI Agent Integration
1. Strategic Alignment
Any AI initiative must start with strategic clarity. Think of the problem you intend to solve with agent integration. Are you aiming to reduce support resolution times, automate routine sales outreach, or surface customized insights that currently live buried in data? Frame your objective in concrete, measurable terms-decrease average first-response time by 40% or improve trial-to-paid conversion by 15%-and secure executive sponsorship by linking those outcomes directly to revenue or cost-savings goals.
Perform a competitive audit: if all major players already offer a generic chatbot, consider a verticalized or data-driven twist that they cannot easily duplicate. For instance, a CRM with industry-specific language models can deliver more precise lead-scoring or email suggestions than a one-size-fits-all assistant.
Finally, clear ROI metrics and experiment frameworks should be established. Define success criteria, activation rates, feature adoption, satisfaction scores, or revenue per account uplift, and use A/B tests or holdout cohorts to measure your AI agent’s true impact. By demonstrating tangible benefits early, you build momentum, secure continued funding, and ensure the AI roadmap remains aligned with shifting market demands.
2. Technical Readiness

Successful SaaS AI agents stand on three pillars: clean data, solid architecture, and operational discipline. Start with a thorough audit of every data source you plan to feed into the model- customer logs, transaction records, support transcripts, and external enrichments. Measure each dataset for completeness, consistency, and labeling accuracy. Any gaps or biases at this stage will be reflected in your agent’s performance, manifesting as incorrect suggestions or a decline in user trust.
Once your data foundation is in place, choose between using third-party AI APIs and hosting an in-house model. Third-party services offer rapid prototyping and sophisticated baselines, but they also introduce ongoing costs, latency considerations, and potential data privacy concerns.
Hosting your model grants total control over custom fine-tuning, version management, and the ability to keep sensitive data behind your firewall, but also demands a mature MLOps setup and computing resources. Whichever path you select, establish clear API specifications, input-validation rules, and response schemas so that every downstream system, from your user interface to analytics pipelines, can ingest outputs reliably.
Scalability and monitoring complete the technical picture. Forecast peak bot usage based on pilot results and secure auto-scaling inference clusters to maintain sub-second response times. Instrument dashboards that track latency, error rates, cost per request, and user feedback signals. With these telemetry streams in hand, your team can detect anomalies early and optimize both model performance and infrastructure spending before issues cascade into production incidents.
3. Business & Go-to-Market
Translating enterprise AI agents into business value requires thoughtful pricing and packaging. Determine whether the agent is a core feature, integral to every license, or a premium add-on that justifies an upsell. Value-based pricing often resonates best: price based on the quantifiable benefits you deliver, such as hours saved or incremental revenue generated. For example, if your AI assistant slashes support costs by 30%, calculate what that saving means per seat or per active user and use it to guide your price tiers and packaging.
Next, build a comprehensive cost model. Beyond development expenses, account for data labeling, ongoing infrastructure (GPU hours, storage), licensing fees, and additional support staff. Compare these outlays against projected revenue lifts, through upsells, churn reduction, or productivity gains, to estimate payback periods. Present these figures transparently in quarterly business reviews to maintain executive backing and secure budget renewals.
Adoption and change management are equally critical. Even the smartest AI agent fails if users don’t embrace it. Embed in-app tutorials, tooltips, and contextual help directly where the agent lives in the UI. Offer customization options, allowing users to adjust the aggressiveness of suggestions or toggle features on and off to accommodate diverse work styles.
Collect real-time feedback through simple prompts (thumbs up/down) on each AI output, and iterate based on that input. Internally, equip your sales and support teams with clear playbooks outlining how to position AI benefits and troubleshoot common concerns. This structured ramp-up ensures that users see immediate value, driving sustainable adoption and unlocking the full potential of your AI investment.
Common Pitfalls of AI Agent Integration & How to Avoid Them

- Context Loss in Conversations: Without a persistent session state, agents forget earlier turns, leading to user frustration. Mitigate this by maintaining dialogue history and injecting relevant context into each model call.
- Hallucinations from Pure Generative Models: Relying solely on a language model risks fabricating facts. Anchor your agent’s responses with retrieved passages from your knowledge base or predefined answer templates for critical interactions.
- Model Drift Over Time: Data distributions evolve, causing once-accurate models to degrade. Implement continuous monitoring of key accuracy metrics and schedule periodic retraining using the latest data.
- Insufficient Human-in-the-Loop Controls: Automating high-stakes actions, billing changes or sensitive disclosures, without manual oversight can spell disaster. Define confidence thresholds that trigger human review and fail-safe escalation paths.
- Technical Debt Accumulation: Fast prototypes can become fragile legacy systems. Invest in MLOps best practices, versioned model registries, infrastructure as code, CI/CD pipelines, to maintain agility and reliability as usage scales.
Conclusion
Integrating an AI agent into your SaaS product is a strategic move that, when executed thoughtfully, can redefine user experience and drive significant business value. By methodically addressing strategic alignment, technical readiness, and business planning and learning from leaders, you position your organization to deliver AI features that customers love and support executives.
Liked what you read?
Subscribe to our newsletter