AI-Powered Sentiment Analysis: Boosting Customer Experience in Digital Age
- Mradul Kanugo
- July 7, 2023
.webp)
Words are powerful and they spread faster than wildfire.
In the business realm, the positive words of a single satisfied customer can influence six or more individuals, whereas an unhappy customer tends to share their negative experience with 9 to 15 people, as per a study. If customers encounter such negative experiences repeatedly, they are likely to cease doing business with you. Consequently, this leads to not only customer loss but also a decline in sales and revenue. Furthermore, negative customer experiences can tarnish your brand reputation and hamper future business prospects.
Harvard Business Review conducted a comprehensive study on the impact of word-of-mouth on customer service. According to their findings, attracting new customers is 5 to 25 times more expensive than retaining existing ones.
Thus, it is important to understand your customers' opinions. Sentiment analysis is a way for this, and artificial intelligence is taking it to the next level. AI sentiment analysis can accurately analyze the emotions of your customers through text-based reviews or tweets which is completely different from traditional sentiment analysis.
Let’s dig deeper and learn what, why, and how of customer sentiment analysis.
Decoding Sentiments: AI vs. Traditional Customer Metrics
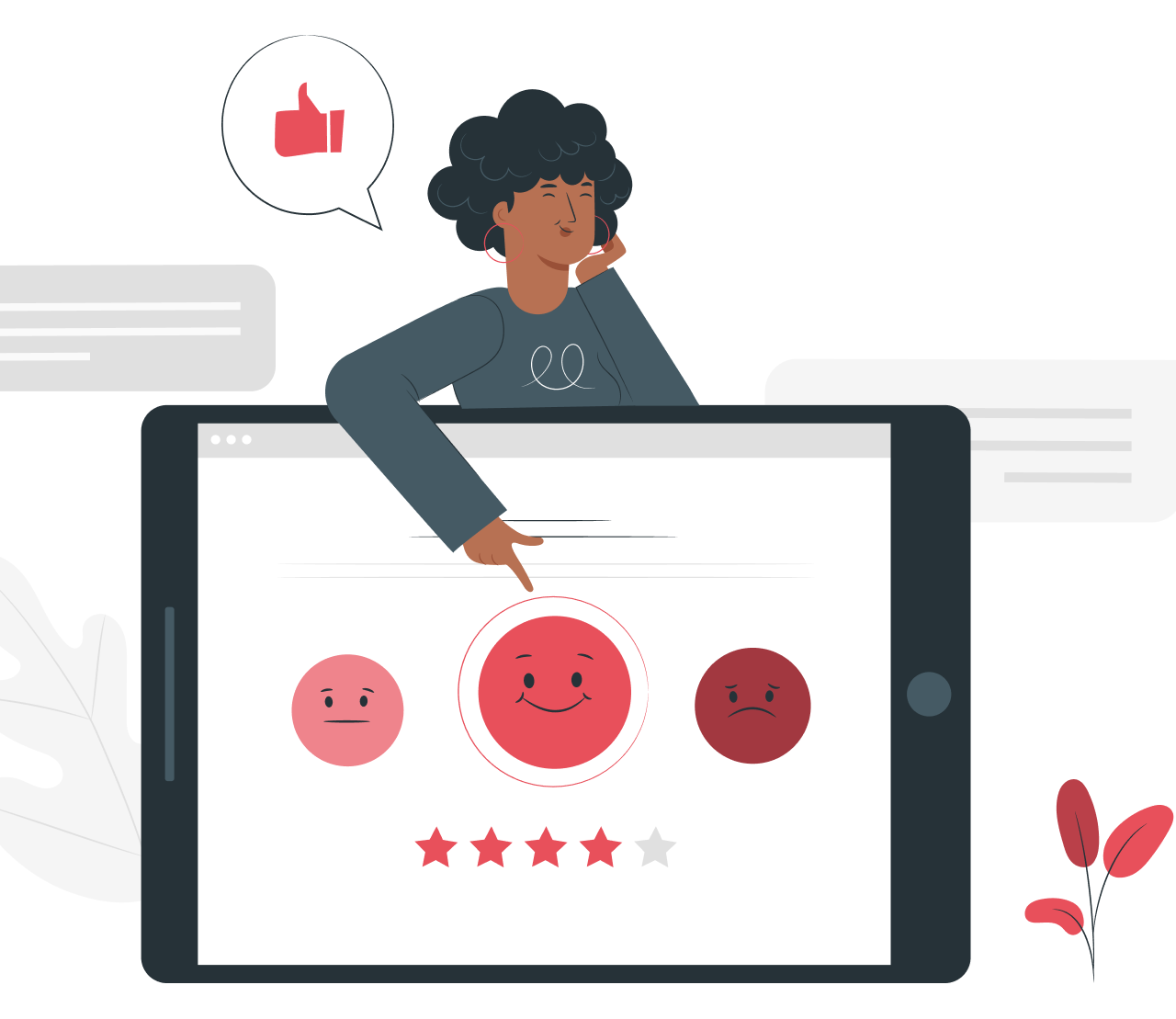
In the field of customer service, customer analytics and sentiment analysis have a key role to play. Now artificial intelligence is bringing a huge shift in this area making it of utmost important for businesses to leverage AI sentiment analysis.
However, it is different than the traditional process of assessing customer experience.
Net Promoter Score
Most of the businesses use net promoter score to identify how many customers are willing to recommend their products/services to other customers. This is basically measured on a scale of 0-10 with range specified for respondents. For example, a customer is put into detractor category if he/she gives the score between 0 and 6. Passives are those who give rate 7 or 8 and customers who rate above 8 are identified as promoters.
Difference between percentage of promoters and detractors is then calculated to find out NPS. However, this approach requires human effort to create and update the sentiment lexicons, and it may not capture the complexities of language, context, sarcasm, or evolving sentiment expressions.
Customer Satisfaction Score
CSAT or customer satisfaction is no different than NPS, but it is also a valuable metric for businesses. Here, the satisfaction is measured through a specific interaction or by asking questions such as “ how satisfied are you with our product?” Customer responses are then recorded on the scale of 1-5 or 1-10. Higher scores indicate greater satisfaction whereas lowest score means unsatisfied customers. The overall CSAT score is the percentage of total number of satisfied responses.
Artificial Intelligence in Sentiment Analysis
AI sentiment analysis leverages advanced machine learning algorithms and natural language processing (NLP) techniques to analyze and interpret sentiment from text-based data in a more sophisticated manner. AI models can be trained on large datasets, enabling them to learn and understand language patterns, context, and even cultural nuances. These models can capture the complexities of human language, including sarcasm, irony, and slang, making them more accurate in sentiment analysis tasks. AI sentiment analysis also has the potential to improve over time as models are continually trained on new data.
From Data to Actionable Insights: The Transformative Benefits of AI Sentiment Analysis
Every feedback is a chance to boost CX. AI sentiment analysis pinpoints the missed loopholes or opportunities for enhancing your product or service to deliver exceptional customer experience.
Here are more benefits of AI sentiment analysis:
Automate Sentiment Analysis
Rather than reading all the customer reviews and feedbacks manually, automate the process through AI-powered sentiment analysis tool. It saves your time and effort on understanding sentiment in large volumes of text or speech data.
Analyze Huge Customer Data
With AI, sentiment analysis can be performed at scale, enabling organizations to process and analyze vast amount of data quickly and efficiently. This is particularly useful in scenarios where there is a high volume of customer feedback, social media posts, or online reviews to analyze.
Strong Customer Relationship
Once a customer, forever a customer is not applicable in this dynamic world. You can lose to your competitors if they treat your customers better than you. Therefore, it is important to prioritize CX and make them feel valued and heard and when you give them what they expect, you win a customer forever. Machine learning-based sentiment analysis is a strategy to achieve this.
Optimized Experience
Great customer service is not simply solving issues through a good product or service. It is about a smooth customer journey. By leveraging artificial intelligence in sentiment analysis, you can identify any hiccups in this journey and fix them before they escalate.
Data-powered Marketing
Rather than wasting your efforts, time, and money blindly on running marketing campaigns, use AI sentiment analysis for targeted marketing. This lets you understand which product features are gaining more attention or resonate with customers’ preferences. How many customers are willing to buy or are there any specific improvements, or expectations they have from future products?
With all these insights, you have valuable data to devise a marketing strategy that will work in your favor.
Brand Reputation Management
By detecting and addressing negative sentiments in real time, you can proactively manage your brand reputation, mitigate potential crises, and engage with customers to resolve issues. Positive sentiment analysis can also be used to identify brand advocates (influencers) and leverage their positive experiences to reinforce the brand's image.
Types of Customer Sentiment Analysis using AI
Sentiment analysis is all about subjective data i.e., users’ feelings and emotions. When combined with artificial intelligence, it is majorly about these main types:
- Aspect-based: Putting it simply, the aspect-based analysis focuses on whether the text is positive, negative, or neutral. This type of analysis is useful for eCommerce, finance, healthcare, and human resources sector.
- Fine-grained: It is a more detailed analysis where the focus lies on emotions, opinions, and intensity.
- Intent-based: As the name suggests, this type of sentiment analysis helps in understanding the intent behind customer interaction. Whether it is a query or complaint, or the customer is expressing a desire. Intent-based sentiment analysis leverages machine learning algorithms.
- Detecting emotion: Are your customers happy, sad, angry, or surprised? Emotion-based analysis helps you understand this through their text or reviews.
- Clause-level: Understanding human emotions is complicated through text or words because of mixed emotions. Read this review for example: “I liked the product though it got delivered late.” Here the customer is happy with the product but complaining about the poor delivery service. This type of mixed review can be identified by clause-level analysis helping you take the right action on negative experience.
Explaining the Mechanics of Sentiment Analysis
Artificial intelligence techniques such as machine learning and natural language processing are the fuel behind customer sentiment analysis. They are either used in combination or alone for better outcomes.
Data Collection: The first step is to gather a dataset of labeled examples. This dataset consists of text documents that are manually annotated with their corresponding sentiment labels (positive, negative, or neutral). The larger and more diverse the dataset, the better the model can learn to recognize different sentiments.
Pre-processing: The collected text data undergoes pre-processing to clean and normalize it. This typically involves removing irrelevant characters, converting text to lowercase, removing stop words (common words like "and" "the," etc.), and handling special cases like stemming or lemmatization to reduce words to their base form.
Feature Extraction: After pre-processing, the text is transformed into a numerical representation that machine learning algorithms can understand. Common techniques for feature extraction in sentiment analysis include bag-of-words (representing each document as a frequency count of words), word embeddings (dense vector representations of words capturing semantic meaning), or more advanced techniques like transformer models (e.g., BERT) that can capture contextual information.
Training a Model: Once the features are extracted, a machine learning model is trained using the labeled dataset. Various algorithms can be used, including Naive Bayes, Support Vector Machines (SVM), Random Forests, or more recently, deep learning approaches like recurrent neural networks (RNNs) or convolutional neural networks (CNNs). The model learns to associate the extracted features with the corresponding sentiment labels.
Model Evaluation: The trained model is evaluated using a separate set of labeled data that was not used during training. This evaluation measures the model's accuracy, precision, recall, F1 score, or other appropriate metrics to assess its performance. If the performance is not satisfactory, the model can be fine-tuned, or the entire process can be iterated.
Sentiment Prediction: The pre-processed text is fed into the model, which outputs the predicted sentiment label (positive, negative, or neutral) based on what it has learned from the training data.
Key Features of AI Sentiment Analysis Tools
- Advanced Natural Language Processing
The AI sentiment analysis tool is able to understand and interpret human language with a high level of sophistication. By being trained on vast amounts of data, the model learns the intricacies of language, including grammar, syntax, semantics, and context. It can grasp subtle nuances, such as sarcasm, irony, and colloquial expressions, allowing it to comprehend text more accurately and generate meaningful responses.
- Real-Time Analysis
A sentiment analysis tool can process large volumes of text data swiftly and provide instant sentiment analysis results. It leverages efficient algorithms and parallel processing capabilities to analyze text in real-time, ensuring that businesses can receive prompt insights and make timely decisions based on the sentiment of their customers or target audience.
- Multilingual Support
It can understand the sentiments expressed in various languages, ensuring that businesses can analyze feedback, reviews, or social media posts from customers worldwide.
- Customizable
Users can define custom categories based on their industry, product, or any other relevant criteria. For example, a hotel chain could define categories such as "room cleanliness," "customer service," or "amenities." The tool then analyzes sentiments within these customized categories, providing more focused insights and allowing businesses to address specific aspects of their products or services.
- Comprehensive Reports
The tool provides detailed reports on sentiment trends, distribution, and key insights. This helps businesses understand overall sentiment, make informed decisions to enhance customer satisfaction, and address issues or opportunities.
Challenges and Limitations
While AI-powered sentiment analysis offers valuable insights, it comes with certain challenges and limitations because of:
- Context and linguistic ambiguity
- The subjective nature of sentiment and individual variability
- Language and cultural biases in training data
- Negation and contextual shifts
- Domain and time sensitivity
- Noisy or unstructured data
Wrapping Up!
Artificial intelligence in sentiment analysis is empowering businesses, particularly those operating in customer-centric domains. It helps you get familiar with your customers, understand their emotions and preferences, and target them strategically.
It is also the cheapest way to retain existing customers and target new ones. However, implementing AI sentiment analysis is not a piece of cake. Softude’s AI and ML experts can make this possible for your business based on your specific requirements.
Liked what you read?
Subscribe to our newsletter