Generative AI Vs Predictive AI: Know The Fundamental Difference Between The Two
- SoftudeOctober 19, 2023
- Last Modified onOctober 4, 2024
Artificial Intelligence is embedding deeper into our daily lives with each passing day it’s hard to ignore it. For businesses starting to leverage AI technologies, Generative AI is the first choice. According to a recent study by Gartner, 45% of businesses are on their way to adopting Generative AI models whereas 10% have already embraced it. Earlier, this figure was only 15%. And then there comes Predictive AI which gives the power of prediction to the businesses. Both are impressive and make a profound impact in almost every sector. But the two are completely different.

In this blog, we will discuss in detail what is the difference between generative AI and predictive AI. So, stay tuned till the end.
What is Generative AI?
Generative AI, also known as Generative Artificial Intelligence, is a fascinating subfield of artificial intelligence that focuses on creating models and systems capable of generating new content that is similar to, or indistinguishable from, human-created content. DALL-E, an AI image generator, and Open AI’s large language model ChatGPT are the first few AI examples that attracted mass interest in Generative AI model.
Models of Generative AI
The heart of Generative AI is generative models, which are algorithms or neural networks
trained to generate data. Some popular generative models include:
Generative Adversarial Networks (GANs): GANs consist of two neural networks, a generator, and a discriminator, which compete against each other. The generator creates fake data, and the discriminator tries to distinguish it from real data. This adversarial training process results in the generator creating increasingly realistic content.
Variational Autoencoders (VAEs): VAEs are probabilistic generative models that encode input data into a lower-dimensional space and then decode it to generate new data samples. They are often used for generating images and have applications in image compression and reconstruction.
Examples
OpenAI's GPT-3 for Text Generation:
OpenAI's Generative Pre-Trained Transformer 3 (GPT-3) is a state-of-the-art language model that can generate coherent and contextually relevant text. It has been used in various applications, from content generation for websites and AI chatbots to language translation and even creative writing. GPT-3 can compose essays, answer questions, and even generate code snippets.
What is Predictive AI?
Its name itself gives a hint of what is predictive AI all about. Like Generative AI, predictive AI is also a subset of AI but differs in capabilities. It focuses on predicting future outcomes based on historical data and patterns and works on models like regression analysis, decision trees, and neural networks to make forecasts or predictions.
Models of Predictive AI
Various models and techniques are employed in predictive AI to analyze data and generate forecasts. Here are some common models used in predictive AI:
Linear Regression:
Linear regression is one of the simplest and most widely used predictive AI models. It models the relationship between a dependent variable (the target) and one or more independent variables (predictors) by fitting a linear equation. It's often used for predicting numerical values and understanding the linear relationship between variables.
Logistic Regression:
Logistic regression is an extension of linear regression used for binary classification problems. It predicts the probability of an observation belonging to one of two classes. It's commonly used in fields like healthcare for disease diagnosis, marketing for customer churn prediction, and credit scoring.
Decision Trees:
Decision trees are a versatile predictive model that uses a tree-like structure to make decisions. Each node represents a feature, and each branch represents a decision based on that feature. Decision trees are used for classification and regression tasks and are known for their interpretability.
Random Forests:
Random Forests are an ensemble learning technique that combines multiple decision trees to improve prediction accuracy and reduce overfitting. They are used in a wide range of applications, including image classification, fraud detection, and recommendation systems.
Neural Networks (Deep Learning):
Deep learning models, particularly neural networks, are powerful predictive AI models that excel in complex tasks such as image recognition, natural language processing, and speech recognition. Recurrent Neural Networks (RNNs) and Convolutional Neural Networks (CNNs) are commonly used architectures for sequence and image data, respectively.
Time series forecasting, support vector machines, K-nearest neighbors, and Bayesian networks are a few more models of predictive AI. They are often used in combination by data scientists and analysts to get accurate predictions as per desired use cases.
Read More: AI-Powered Sentiment Analysis: Boosting Customer Experience in Digital Age
Examples: AI Credit Scoring
One of the major areas of application of predictive AI is in assessing the creditworthiness of buyers. In financial institutions, this process is of utmost importance to reduce the risks that come with lending loans to fraud customers. However, the manual way is very lengthy and inefficient thus putting institutions at a great risk.
Predictive AI is making this process not only automated but also accurate through machine learning algorithms. These algorithms are capable of organizing tons of data points in real time and give patterns that show whether a particular buyer can repay the loan or not. Additionally, these models analyze other indicators such as current income, potential ability to earn, and many more for precise profit predictions. This is just one example of predictive AI.
Generative AI vs Predictive AI: 4 Major Difference Between These Types of AI
Majorly, the two differs in terms of training data and the outputs they produce. Refer to the table below for an overview of the key areas of comparison in Generative AI vs Predictive AI.
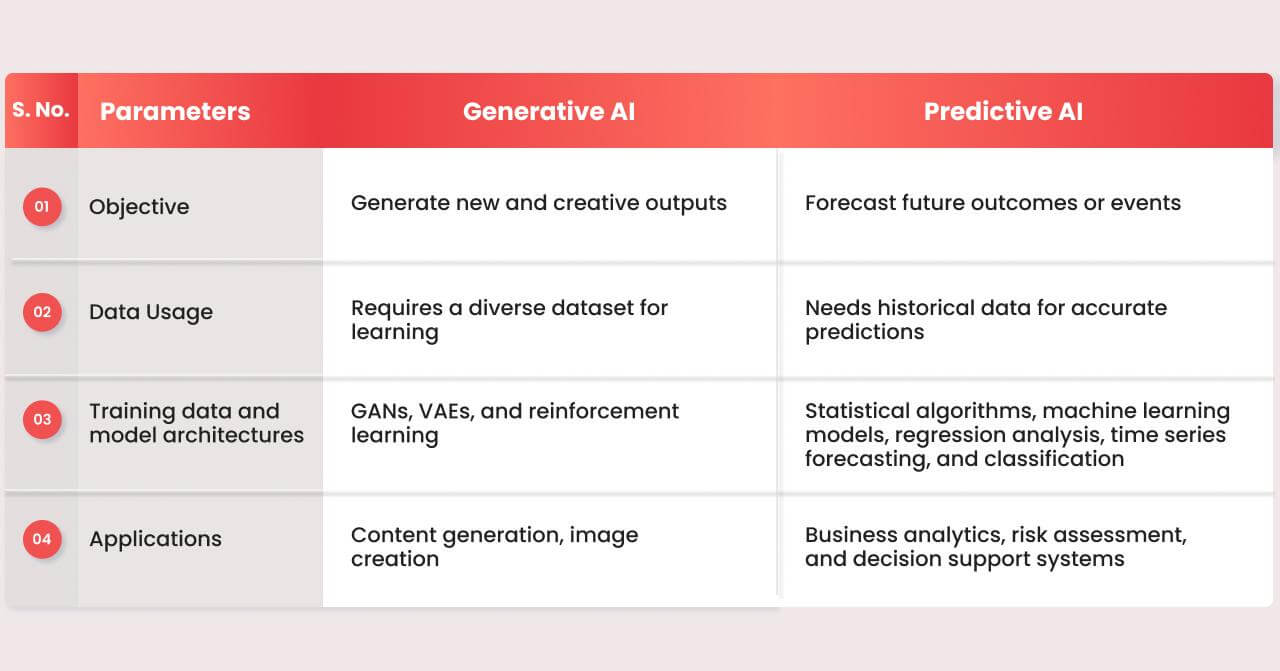
Undoubtedly, both have impressive capabilities, but they are not without limitations. Generative AI may not always give creative outputs that meet users’ expectations. It might be due to the limited data or poor-quality dataset in which it is trained. Another challenge is they are resource-intensive and require huge computational resources which increases the cost of training these models. And then there are ethical and social considerations. In the case of predictive AI, the quality of data matters a lot as incomplete, biased, or insufficient data will not generate accurate predictions.
Liked what you read?
Subscribe to our newsletter